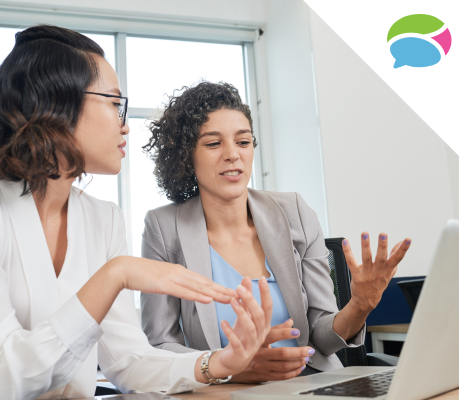

SmartDataLake: Improving data lakes through better data management, early insights and visual analytics
Data, without context and purpose, is largely without value, sheer rows and columns of characters and numbers and attributes. Data lakes, combine data from arbitrary sources, and pool them up in one big lake of bits and bytes, different formats, sizes and of largely varying quality. SmartDataLake, on the other hand, provides the means and the tools to find the needle in the hay stack and convert data, into information.
While the overall research strategy and goals have been rather well-defined from the very beginning of the project, a strong focus has been put on shaping the details and steering the implementation of the various components. Firstly, the overarching goals have been classified into five key challenges: handling data heterogeneity, reducing storage, making sense of the data, monitoring changes and, last but not least, keeping the human in the loop during these steps. These goals have then been derived to specific challenges, derived by both the research and industry partners, into specific use cases, as outlined in the following.
Efficient data storage and management
Handling data is managed and improved through storage tiering for historical data, where infrequently used data may be autonomously assigned to cheaper cold storages, as well as providing data virtualization functionality, that essentially enables the user to work directly on heterogenous data types and formats and to use a tool that they are already familiar with.
Scalable data preparation and analytics
Regarding making sense of the data, SmartDataLake defines a set of methodologies that facilitate typical data processing techniques but are optimized for large data sets. These include assembling entity profiles, e.g., where an entity’s attributes may be merged across different datasets, through applying entity resolution, computing and presenting descriptive attributes for the combined dataset, and then identifying similar entities for applications that require e.g., providing alternative opportunities or identifying potential, promising entities. As a related activity, one of the components focuses is to accurately predict a potential relation between two entities by looking at the relations of the given entity rather than comparing the specific attributes with regard to their similarity. The application of comparing entities based on their similarity is applied on both the individual attributes of the entity, such as their classifications or tags, their numerical values, such as the number of employees in a given organisation, as well as the geographical location, and secondly on their timely properties, e.g., when the data is provided as a time series, though time series forecasting and detection and correlation of different time series in order to support finding and identifying seasonal patterns.
SmartDataLake further provides high-level analytics functionality, which builds on the previously defined components, in order to convert the previously created rich sets of data into actionable information. These use-cases consist of ranking of different types of entities, in order to identify the most prominent ones, community detection for identifying groups of entities with a strong bond as well as detecting changes in the data, which may be used for identifying outliers and anomalies.
Visual analytics and intuitive insights
Finally, in a strive to keep the user in the loop, the primary use-cases that are in focus, and reflect the previously specified use-cases, facilitate the visual representation of the various individual steps along the processing pipeline, e.g., presentation of the computed attributes and presenting the outcomes and results of the individual components, through e.g., visual analytics of time series, data profiling and descriptive statistics, providing insights into the considered data, and predictive parameter based visualisations, that show insights into how changing a parameter would change the outcomes by showing the incremental changes side by side.
In summary, SmartDataLake makes working with heterogenous datasets, and thus gaining early insights, more efficient, faster, and, not least, smarter.
Keywords
Big data, data lake, smart, data analytics, data visualization, insights, data driven